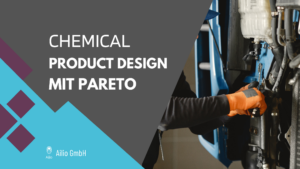
Chemical Product Design with Pareto Optimization: An innovative approach
In the chemical industry, the development of new products is at the heart of technological progress. A key method in this area is Chemical Product
Data science projects can be very challenging. The basic challenge is often not that they are particularly complex, costly or lengthy, as is the case with most software projects, but rather in the minds of the managers.
As a service provider whose mission is to bring data science and artificial intelligence to medium-sized companies and to exploit the untapped potential, we on the Ailio side are often faced with a very specific challenge:
“We’re developing something with technologies that are abstract to you where we can’t promise you the exact outcome and whether the challenge is even solvable.”
This doesn’t sound like a very management-friendly pitch at first, and of course we’ll do our best to make it sexier and more comprehensible.
In essence, however, the statement is true – Data Science projects always have a Research and Development (R&D) character. Every company is different – even if they come from the same industry and have a similar data and IT strategy. The quality, quantity and form of the data is always different and has a massive impact on approach, effort as well as success.
In short, you usually just don’t know beforehand and you just have to do it. As a rule, good results can be achieved with a manageable amount of effort, the amount of data available to an SME is completely sufficient for most use cases, and with a good evaluation, the use case usually does not fundamentally fail.
But you just don’t know beforehand … and false promises have a taint as they would say in southern Germany.
So how can we ensure that projects are a success and make the investment decision as attractive as possible?
Basically, it is recommended to think in small risk-minimizing steps and not to try to plan the big picture immediately. You have an exciting use case and data for it? First a deep dive into the data to really understand it, get questions answered, see what is missing and needs to be expanded … this usually takes a week at most and has many tangible benefits. Starting with the company understanding its own data in the first place.
After that, a short proof of concept is recommended in which a minimal version is implemented that proves that the basic assumption is correct and feasible. If the deep dive reveals that the data structure and infrastructure first have to-dos to enable the company to execute DS projects … this is still necessary before PoC as needed.
A proof of concept should ideally take between 5 and 20 days, depending on the use case, and thus keep the investment required for it lean.
At the end of these phases, you have invested about 15-30 project days, which is a manageable investment for most companies, with the result of having a clear picture of your own data situation, having it transformed and optimized, and knowing exactly what can be implemented with it and to what extent.
Based on the PoC findings, a concrete project plan for the MvP can usually be created and more concrete promises can be made … so we are slowly becoming management-ready. From here on we have a normal software project.
Many data science projects are actually doomed before they are even started. Especially in the corporate environment, you wouldn’t believe how much we have already developed that, although it was successful and would have been very useful to use, never exceeded the PoC phase.
Why is that? In the end, data science projects change the daily work of people who are often never consulted or involved in the development. As a result, the initial good idea was never advertised and accepted internally. Here comes the unpleasant surprise at the end, when the person in charge of the department the project is supposed to help is not really behind it and the employees do not understand what it is all about or are even afraid of it.
The same game as in point 2, but on a technical level … a company is able to massively increase the costs for a data science project for itself and at the same time minimize the probability of success by not giving the data science team the access and support it would need due to departmental thinking and a lack of willingness to cooperate on the part of corporate IT. A DS project rarely works by itself. Often the results need to be integrated into the software, API’s need to be addressed, data needs to be made available and explained, or perhaps captured in the first place.
Believing in data science is one thing … you can smile at it or take it seriously. In the end, the goal of data science projects is to make data-driven and fact-based decisions. You develop a software that sees all the data, recognizes it and can make connections that the human brain can never oversee or understand. In the end, the decision how to deal with the results of the software is often still in human hands. In the end, data science has little to do with faith as it is the way away from manual work and gut feeling decision to automation and fact-based approach. Here, there needs to be a shift in thinking at the organizational level to understand – that even if the results are not calculable and predictable – they will certainly be more concrete and reliable than pure gut feeling. An understandable thought in itself, but one that nevertheless causes many inexperienced decision-makers in the data science environment a stomachache when making investment decisions.
Ailio GmbH is a Bielefeld-based service provider specializing in data science and artificial intelligence. We advise in both areas and unleash the potential of data that is currently lying fallow in German SMEs. In doing so, we take a cost-optimizing and risk-minimizing approach. If you are interested, please contact us directly!
In the chemical industry, the development of new products is at the heart of technological progress. A key method in this area is Chemical Product
In the world of data analysis and big data management, Azure Synapse Analytics and Databricks are two prominent names. Both offer powerful tools for processing
The implementation of Databricks, a leading platform for big data analytics and artificial intelligence, is a crucial step for companies looking to improve their data